Connecting Institutions to Build Flagship Dataset for Healthcare AI
With support from a recent National Institutes of Health U01 grant, principal investigator Yuan Luo, PhD looks to further integrate artificial intelligence with clinical medical practice.
“These days there's a lot of hype about AI, and frankly there’s also quite a lot of success in AI in general,” says Luo, Chief AI Officer at the Northwestern University Clinical and Translational Sciences (NUCATS) Institute and Institute for Artificial Intelligence in Medicine. “But such successes have barely, if at all, translated to the healthcare setting.”
The project involves Northwestern University, MIT, and multiple Clinical and Translational Science Award (CTSA) hubs, including those at Tufts University, Washington University, and the University of Alabama at Birmingham.
“We're covering a diverse geographical region, which is important not only because it captures diverse patients in diverse areas, but also because practice differs at these sites,” says Luo.
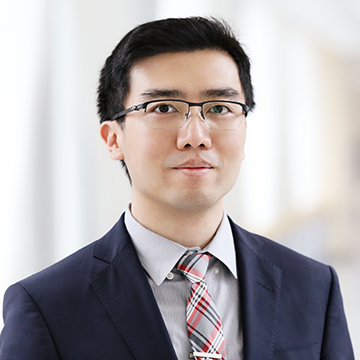
We're covering a diverse geographical region, which is important not only because it captures diverse patients in diverse areas, but also because practice differs at these sites.”
The first aim of the project is to construct a diverse dataset of patient profiles across the four CTSA locations. With the combination of adult and pediatric ICU patients, Luo estimates that the database will capture around 500,000 profiles.
“We can create a flagship dataset that is large enough and diverse enough to capture all aspects of the patient profiles, before and after their ICU stay,” says Luo. “That will make these datasets really useful in terms of training a model to predict a patient's outcome or training a model to recognize certain less frequent diseases, for example.”
The researchers will then develop an advanced AI algorithm, which will be trained on the dataset. Luo compared the algorithm to doctors drawing on past experiences, including their medical school training, when diagnosing a patient. The algorithm will store patient information in a memory network and use that information when seeing patients in the future with similar profiles. This is why including a diverse set of patients from different regions helps the algorithm work best.
“Having a culturally diverse dataset is important because, if you just train an AI algorithm and apply it blindly to all people, research has shown that those AI algorithms will disproportionately misrepresent minority groups.” says Luo.
Having a comprehensive profile on patients, including their background and the care they have received in the past, will inform the algorithm’s ability to help in clinical settings.
“Nowadays, people are talking about multi-model datasets, meaning that you're not only going to have access to the patient's EHR data, you're also going to have access to their imaging, such as CTs or MRIs,” says Luo. “And you also, in many cases, have access to their genetic sequencing data. Once you have all the data, the varying perspectives will help you triangulate the evidence to look at the possible mechanisms of the patient's pathophysiology progression and how to most effectively intervene.”
Part of the $5 million grant (U01 TR003528-01A1) will serve to streamline patients’ raw data so the information can be consumed by an algorithm.
“Another part of the funding will go to creating this open source repository and then creating training materials to advance the next generation of AI medical researchers,” says Luo.
He adds that there are considerable barriers to translating the success of AI to the clinical domain. Education and overall data accessibility comprise an important piece of the project.
“Part of the challenge is that we need a much larger and diverse research workforce. This grant, by creating the dataset and creating the methodology, will also help train the central workforce,” says Luo. “We can all work on a shared dataset, and the methods that we develop will be open sourced and released to the community so that they can benefit all researchers.”
In order for this work to truly flourish though, Luo hopes to create a larger consortium with other CTSA hubs across the country.
“Each CTSA hubs captures a specific portion of the diverse racial and ethnic profiles of the nation,” he explains. “And once we have all the pieces of the puzzle, we can have a holistic view of the issue and then develop an optimized algorithm for addressing critical issues such as healthcare disparities.”
Luo believes that widescale collaboration across medical institutions is the key to making this project both successful and sustainable. He used the term “Industrial AI” to describe the future of this growing field.
“Before the Industrial Revolution, it was boutique crafting that made production goods. But after the Industrial Revolution, things could be streamlined, things could be standardized. And part of the standardization is that we have a larger diverse workforce so we can scale up,” says Luo. “We're hoping this could be among the steps towards an Industrialized AI revolution so that we can increase the productivity and efficiency of training those algorithms and their deployments.”
Written by Olivia Lloyd